AI Document Processing: How AI Is Making Manual Data Entry Obsolete
AI document processing has evolved from a nice-to-have feature to a business-critical capability. This article explores how modern AI-native solutions are eliminating manual data entry, achieving 95-99% accuracy, and delivering dramatic ROI by understanding documents the way humans do. Learn how companies across industries are implementing this technology to save thousands of hours and transform their operations.
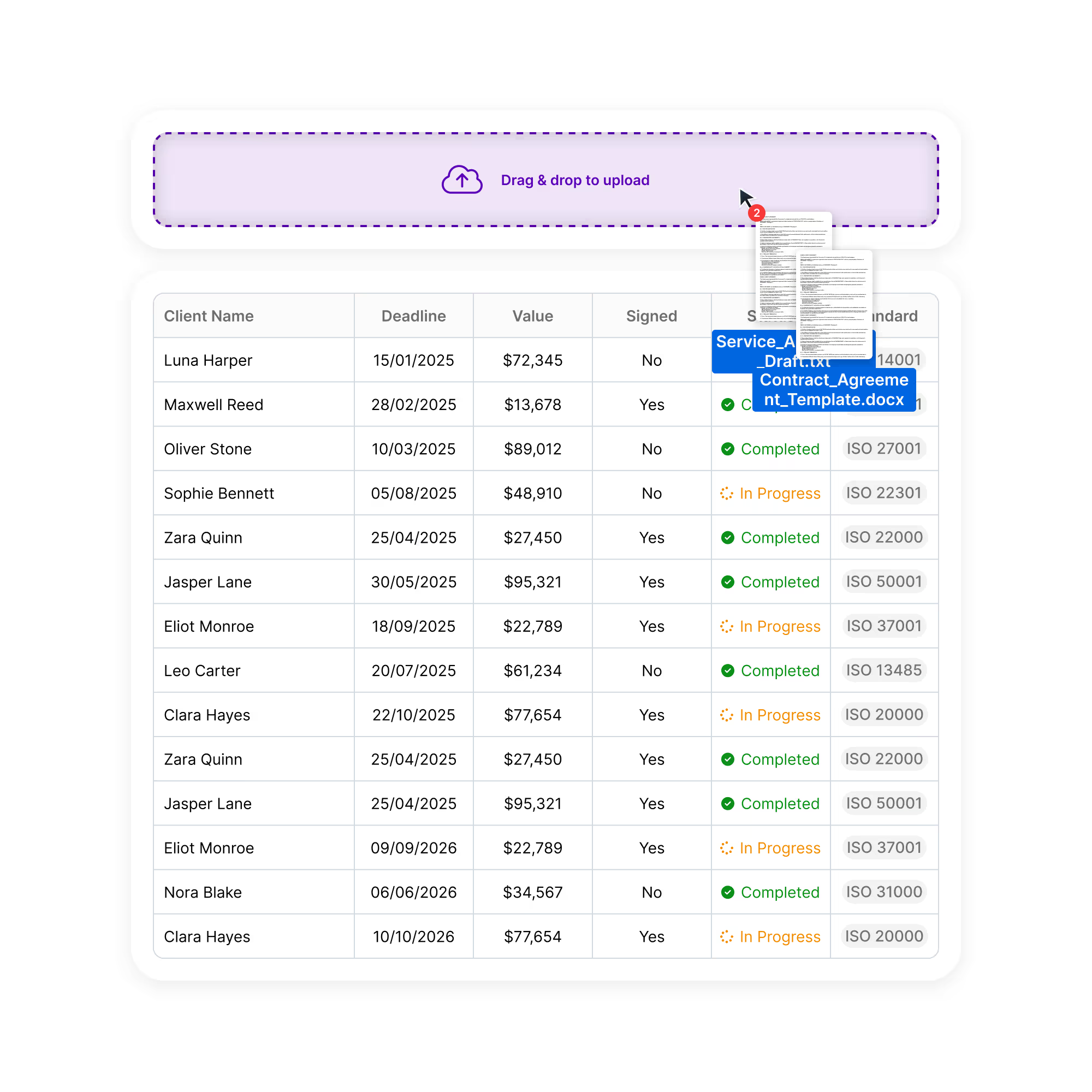
Most companies are still paying humans to manually extract data from documents in 2025. I'm talking about armies of people typing information from invoices, contracts, and forms into various systems - work that's mind-numbingly boring, error-prone, and incredibly expensive.
Yet here we are, with businesses spending billions on this digital equivalent of moving rocks from one pile to another. Why? Because until recently, the alternatives weren't much better.
Traditional document processing solutions promised automation but delivered headaches: rigid templates that break when a form changes slightly, complex rules that require constant updating, and accuracy rates that make even the most patient operations leaders pull their hair out.
But there's been a fundamental shift in what's possible. AI document processing has evolved from a nice-to-have tech toy to a business-critical capability that's reshaping how organizations handle information.
In this article, I'll break down exactly how AI document processing works in 2025, why it's finally ready for prime time, and the shocking ROI numbers companies are seeing when they implement it correctly.
The Problem with Traditional Document Processing
Let's talk about what document processing actually looks like at most companies today:
- The manual approach: Employees manually type data from documents into systems like ERP, CRM, or accounting software. It's slow, error-prone, and soul-crushing work. According to McKinsey, employees spend 30% of their time on these repetitive document tasks.
- Template-based OCR: Slightly better, but still problematic. These systems work only if documents follow exact templates. One field moves a millimeter? The system breaks. New supplier with a different invoice format? Back to manual processing.
- Rules-based extraction: More flexible than templates, but requires extensive configuration. IT teams spend months creating rules for every possible document variation. When documents change (as they always do), the rules need updating.
All these approaches share the same fundamental problems:
- Rigidity: They can't handle variations or new document types without significant rework
- High maintenance: They require constant updates as documents evolve
- Limited accuracy: Even the best systems miss critical information or extract it incorrectly
- Scaling issues: The more document types you add, the more complex and brittle the system becomes
This explains why, despite decades of attempts to automate document processing, most businesses still rely heavily on manual work. The technology simply wasn't good enough to handle the messy reality of business documents.
But that's all changed.
How AI Document Processing Actually Works
Modern AI document processing is fundamentally different from traditional approaches. Instead of rigid templates or brittle rules, it uses a combination of technologies to understand documents the way humans do:
1. Computer Vision + OCR
First, the system needs to see the document. Modern computer vision combines traditional OCR (optical character recognition) with deep learning to:
- Identify document types automatically (Is this an invoice? A contract? A form?)
- Handle poor quality scans, phone photos, and distorted images
- Recognize both printed and handwritten text
- Understand document structure (headers, tables, footers, etc.)
The accuracy improvements are staggering - we're talking 99%+ accuracy on text recognition compared to 80-90% with traditional OCR.
AI-native platforms like Lleverage don't just "read" text like traditional OCR systems – they actually understand what they're seeing, just like a human would. This contextual understanding makes all the difference in processing accuracy and adaptability.
2. Natural Language Processing (NLP)
Once the system sees the text, it needs to understand it. That's where NLP comes in:
- Entity recognition: Identifying what type of information a piece of text represents (dates, amounts, account numbers, etc.)
- Relationship mapping: Understanding how different pieces of information relate to each other
- Context understanding: Recognizing that "due date" and "payment terms" might refer to the same concept
3. Intelligent Extraction
With understanding comes extraction. Modern systems can:
- Pull structured data from unstructured documents
- Extract line items from tables with complex formats
- Identify critical clauses in contracts
- Validate extracted information against business rules
4. Continuous Learning
The real magic happens over time. Unlike traditional systems that remain static, AI document processing gets better with use:
- Learning from corrections and feedback
- Adapting to new document formats automatically
- Improving accuracy across documents over time
- Transferring knowledge from one document type to another
This learning capability means ROI actually improves over time - the opposite of traditional tech investments that depreciate.
Real-World Applications Across Industries
The impact of AI document processing varies by industry, but the pattern is the same: massive efficiency gains, error reduction, and new capabilities that weren't possible before.
Finance & Accounting
Invoice Processing
- Before: 15-20 minutes per invoice with manual processing
- After: 30 seconds per invoice with AI processing, 90% fully automated
Financial Document Analysis
- Automatically extracting data from bank statements, tax forms, and financial reports
- Reconciling information across multiple sources
- Flagging anomalies and potential compliance issues
One accounts payable team at a manufacturing company was processing 15,000 invoices monthly with a team of 12. After implementing Lleverage's AI document processing, they handle the same volume with just 3 people while improving accuracy and cutting processing time from days to hours.
Legal
Contract Analysis
- Extracting key clauses, obligations, and rights
- Comparing terms against standard templates
- Identifying missing clauses and potential risks
Legal Document Review
- Processing discovery documents in litigation
- Extracting relevant facts and precedents
- Organizing evidence by relevance
A law firm reduced contract review time by 60% using AI document processing to extract and analyze key provisions, allowing attorneys to focus on strategic advice rather than document scrutiny.
Healthcare
Patient Records
- Digitizing and structuring handwritten notes
- Extracting diagnosis codes and treatment plans
- Identifying medication information and dosages
Insurance Claims
- Processing claims forms and supporting documentation
- Extracting procedure codes and coverage details
- Identifying missing information that could delay processing
A healthcare provider reduced insurance claim processing time from 7 days to less than 24 hours by automating document intake and information extraction, resulting in faster reimbursements and improved cash flow.
Real Estate & Mortgage
Loan Document Processing
- Extracting borrower information from application forms
- Analyzing income verification documents
- Processing property appraisals and inspection reports
Property Management
- Processing lease agreements and amendments
- Extracting key dates, terms, and obligations
- Managing property inspection reports
A mortgage processor increased application throughput by 300% while reducing errors by 80%, allowing them to process more loans with the same staff during a high-volume period.
The Business Case: ROI That's Too Good to Ignore
The business case for AI document processing is compelling across multiple dimensions:
1. Direct Cost Savings
- Labor reduction: 60-80% decrease in document processing staff requirements
- Error reduction: 80-95% fewer errors, avoiding costly mistakes and rework
- Processing costs: 50-70% reduction in cost per document processed
2. Productivity Gains
- Processing speed: 10-100x faster document processing
- Higher throughput: Handle more volume without adding staff
- Scalability: Process spikes in volume without hiring temporary workers
3. Strategic Benefits
- Data visibility: Extract insights from documents that were previously inaccessible
- Improved compliance: Better documentation and audit trails
- Enhanced customer experience: Faster response times and fewer errors
Real ROI Example
Let's look at a mid-sized retail company processing 5,000 supplier invoices monthly:
Before AI Implementation:
- 4 full-time employees at $60,000/year each: $240,000
- Average processing time: 15 minutes per invoice
- Error rate: 7% (350 invoices with issues monthly)
- Error correction cost: $50 per error = $210,000 annually
After AI Implementation:
- 1 full-time employee for exception handling: $60,000
- Software cost: $50,000 annually
- Average processing time: 45 seconds per invoice
- Error rate: 0.5% (25 invoices with issues monthly)
- Error correction cost: $15,000 annually
Annual Savings:
- Labor: $180,000
- Error reduction: $195,000
- Total savings: $375,000
- ROI: 375%
And that's not counting soft benefits like faster processing times, better supplier relationships, and freed capacity for strategic work.
Implementation Guide: Getting Started Without the Pain
The benefits are clear, but implementation can be daunting. Here's a practical approach that works:
1. Start With a Focused Use Case
Don't try to automate everything at once. Pick a document type that is:
- High volume
- Standardized enough to be automatable
- Currently causing pain in your organization
- Has clear ROI potential
Invoice processing, standard contracts, and application forms are common starting points with proven success rates.
2. Choose the Right Technology Approach
You have several options:
- Build: Create custom document processing using ML frameworks (high effort, high customization)
- Buy: Use specialized document processing software (medium effort, medium customization)
- Platform: Use an AI-native platform that includes document processing capabilities (low effort, high flexibility)
For most companies, the AI-native platform approach offers the best balance of speed, cost, and capabilities. Lleverage stands out here with its true AI-native architecture that includes built-in vector databases and RAG (Retrieval Augmented Generation) pipelines that ensure superior output quality at scale. This approach enables document processing that not only extracts data but actually understands the content, allowing for more intelligent automation and higher accuracy rates than traditional solutions.
3. Prepare Your Data
The quality of your implementation depends on the data you use:
- Collect sample documents covering various formats and edge cases
- Include examples of problematic documents that cause issues today
- Organize documents by type and subtype
- Document current manual processes for validation purposes
The more diverse your sample set, the better your AI will perform across different document variations.
4. Implement With a Hybrid Approach
The most successful implementations use a human-in-the-loop approach:
- Start with a subset of documents to build confidence
- Review AI extractions before pushing to downstream systems
- Use human feedback to improve model performance
- Gradually increase automation as accuracy improves
This approach builds trust while ensuring you don't introduce new errors into your processes.
5. Expand and Optimize
Once your initial use case is successful:
- Add more document types to the automation
- Integrate with additional systems
- Implement straight-through processing for high-confidence documents
- Train your team to handle exceptions rather than routine processing
Each expansion multiplies your ROI while building on your initial success.
Common Objections (And Why They Don't Hold Water)
Despite the clear benefits, some organizations hesitate to implement AI document processing. Let's address the most common objections:
"Our documents are too complex/unique/variable"
Reality: Modern AI document processing is designed for complexity and variation. Unlike template-based systems, AI can learn from examples and adapt to new formats. The more variable your documents, the more value AI brings compared to rule-based systems.
"We tried OCR before and it didn't work"
Reality: Comparing traditional OCR to modern AI document processing is like comparing a bicycle to a Tesla. The fundamental technology has evolved dramatically, with accuracy improvements of 50-200% depending on the document type.
"It's too expensive to implement"
Reality: Cloud-based AI-native platforms like Lleverage have dramatically reduced implementation costs. Most organizations achieve positive ROI within 3-6 months. Additionally, starting with focused use cases allows you to prove value before expanding.
"Our compliance/regulatory requirements won't allow it"
Reality: AI document processing often improves compliance by creating consistent, auditable extraction processes. Modern systems provide detailed audit trails showing exactly what was extracted and with what confidence level. This is where true AI-native automation outperforms traditional automation – the ability to handle nuanced compliance requirements while maintaining auditability.
"We need 100% accuracy, and AI isn't there yet"
Reality: Even human processing isn't 100% accurate - typical manual error rates range from 5-15%. AI document processing routinely achieves 95-99% accuracy, and uncertain extractions can be flagged for human review. The hybrid approach gives you both efficiency and accuracy.
The Future of Document Processing
The AI document processing landscape continues to evolve rapidly. Here are the trends shaping its future:
1. Multimodal Understanding
Next-generation systems will understand not just text, but also:
- Images and diagrams within documents
- Document layout and design elements
- Handwritten annotations and signatures
- Visual elements like charts and graphs
This holistic understanding will enable even more complex document types to be automated.
2. Autonomous Document Agents
Rather than just extracting information, document agents will:
- Take action based on document content
- Route documents to appropriate workflows
- Request missing information automatically
- Negotiate terms and suggest amendments
This moves beyond processing to autonomous document handling that mimics human capabilities. The difference between AI agents and AI workflows becomes crucial here - while workflows follow predefined paths with AI enhancement, agents can operate independently to achieve document-related goals.
3. Knowledge Extraction and Synthesis
Future systems will not just extract data but understand it:
- Connecting information across multiple documents
- Building knowledge graphs from document collections
- Identifying trends and patterns in document data
- Generating insights and recommendations
This transforms document processing from a cost center to a strategic capability that drives business value.
4. Self-Designing Automation
The ultimate evolution is systems that:
- Analyze document workflows without human input
- Identify automation opportunities automatically
- Design and implement extraction models
- Continuously optimize performance without human intervention
This "automation of automation" will dramatically reduce the effort required to implement and maintain document processing systems.
FAQ: AI Document Processing
How accurate is AI document processing compared to humans?
Modern AI document processing systems regularly achieve 95-99% accuracy on well-defined fields, compared to human accuracy rates of 90-95%. More importantly, AI is consistent - it doesn't get tired, distracted, or make random errors. For critical fields, human verification can be added to ensure 100% accuracy where required.
How long does implementation typically take?
Implementation timelines have shortened dramatically with modern AI platforms. Simple document types can be automated in as little as 1-2 weeks. More complex implementations covering multiple document types might take 4-8 weeks. This is a dramatic improvement over traditional approaches that often required 6-12 months of development.
What kind of documents work best with AI processing?
While AI can handle a wide range of documents, the best candidates for initial implementation are:
- High-volume, standardized documents (invoices, applications, forms)
- Documents with structured or semi-structured formats
- Documents that follow industry conventions
- Documents where manual processing creates bottlenecks
As you gain experience and the AI improves, you can move to more complex document types.
How does AI document processing handle sensitive information?
Modern AI document processing platforms include robust security features:
- Data encryption in transit and at rest
- Role-based access controls
- Audit trails for all processing activities
- Redaction capabilities for sensitive information
- Compliance with regulations like GDPR, HIPAA, etc.
Many platforms also offer on-premises or private cloud deployment for organizations with strict data sovereignty requirements.
What's the difference between document processing and RPA?
Robotic Process Automation (RPA) mimics human actions in digital systems, essentially creating digital robots that click buttons and enter data. Document processing is about extracting meaning from unstructured data like documents, images, and text. The two technologies are complementary - document processing extracts the data, and RPA can use that data to update systems.
Modern AI-native platforms like Lleverage combine both capabilities for end-to-end automation, allowing businesses to create comprehensive solutions that handle everything from document intake to data extraction to system updates – all without writing a single line of code. This integrated approach delivers significantly higher ROI than piecing together separate systems.
Can AI document processing integrate with our existing systems?
Yes, modern document processing solutions are designed for integration. They typically offer:
- APIs for programmatic access
- Pre-built connectors for common business systems (like Lleverage's 2,000+ integrations)
- Webhook capabilities for event-driven integration
- Export formats compatible with various data systems
This flexibility allows document processing to fit into your existing technology ecosystem without major changes.
The shift to AI document processing represents one of the highest-ROI opportunities for businesses today. It transforms a tedious, error-prone process into a strategic advantage, freeing human talent for more valuable work while improving accuracy and speed.
As the technology continues to mature, the gap between organizations that embrace AI document processing and those that stick with manual methods will only widen. The question isn't whether to automate document processing, but how quickly you can implement it to capture the competitive advantage it offers.
Ready to explore how AI document processing could transform your operations? Book a demo with our team to see how Lleverage's AI automation platform can streamline your document workflows.